
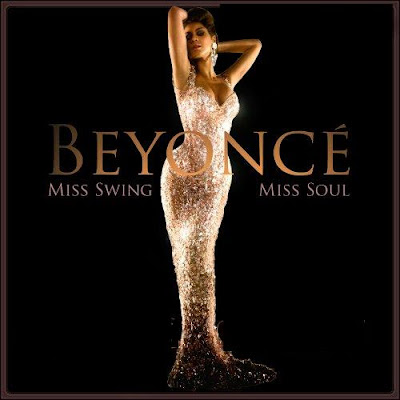
Addressing the rapid increase in swing rate on the lower end of the spectrum, I would imagine that many of these pitches are thrown in advantageous counts from the perspective of the pitcher - two-strike counts. Instead, the choice depends on the pitch type and what the hitter is guessing or picks up out of the pitcher’s hand. My hunch is that once a pitch reaches a certain threshold of competitiveness (in terms of challenging the hitter to swing), the swing decision is not as tethered to the chance of the pitch being called a strike. Intuitively, you would expect this relationship to be linear throughout the probability interval for every 1% increase in called strike probability, the swing rate would also increase by some corresponding percent described by the slope of a line regardless of where you are along this interval. For the more competitive pitches, the changes in swing rate are much smaller. Swing rates increase rapidly as the called strike probability approaches 0 and 100%. The following represents the swing rates in each of those bins: I binned every pitch in increments of 10% of called strike probability.
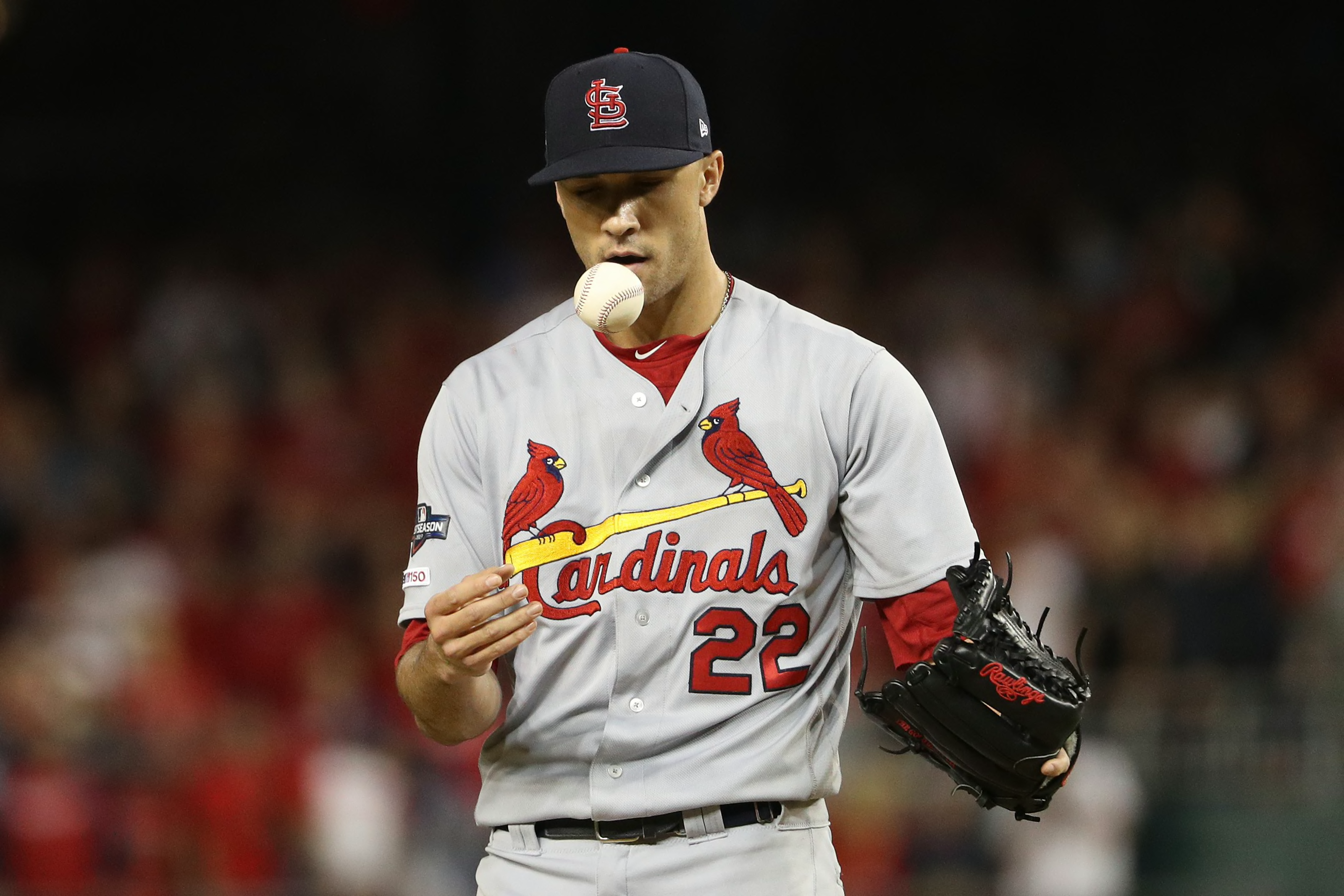
And pitches with expected probabilities closer to zero are nowhere near the strike zone. Pitches at the edges of the zone have anywhere from a 40–60% chance of being called a strike. Pitches with higher probabilities of being a called strike if taken are toward the heart of the zone. I applied the model to all pitches from the 2019 and ’20 regular seasons, which yielded the probability of a called strike on every pitch. For the test set, the model was about 92.5% accurate, in that it correctly predicted whether a pitch was called strike 92.5% of the time. The model was trained on 80% of pitches called a ball or strike from the 2020 season, with the remaining 20% used as the test set. My model was trivial (relative to the research I linked above) in that I just considered pitch location and pitch movement for the purpose of this exercise, I thought that would be enough to get the idea across. Similar to how pitches are evaluated for the purpose of studying catcher framing, I created a general additive model for gauging the probability that a given pitch would be called a strike. One method we can use is to group pitches by their probability of being called a strike. But in smaller samples, the lack of distinction between pitches and their proximity to the strike zone makes judging a player’s swing decisions difficult. Granted, this distinction lacks meaning over many pitches selective hitters with elite batting eyes will separate from their less fastidious peers with respect to chase rate over time. A lot of swing decision analysis is done in the binary, but as many analysts have shown, looking at the gradations in the strike zone can be revealing. I have a bone to pick, though: there is often no differentiation between pitches that just miss the defined strike zone versus those that miss by multiple feet, or pitches that just nick the strike zone as opposed to pitches right down the middle. With a sufficient sample, these binary classifications give us insight into how players approach their plate appearances relative to their peers, which hitters are better at discerning the strike zone and which are more aggressive. Over the course of hundreds or thousands of pitches, this provides an easy-to-comprehend method of effectively evaluating a player’s approach. We consider whether the pitch was in the strike zone (as defined by your data provider of choice) and whether the batter swung. Swing decisions are generally evaluated with limited nuance.
